Ganymede Bio and Kytopen to bring cloud-native structured data to gene-modified cell therapy
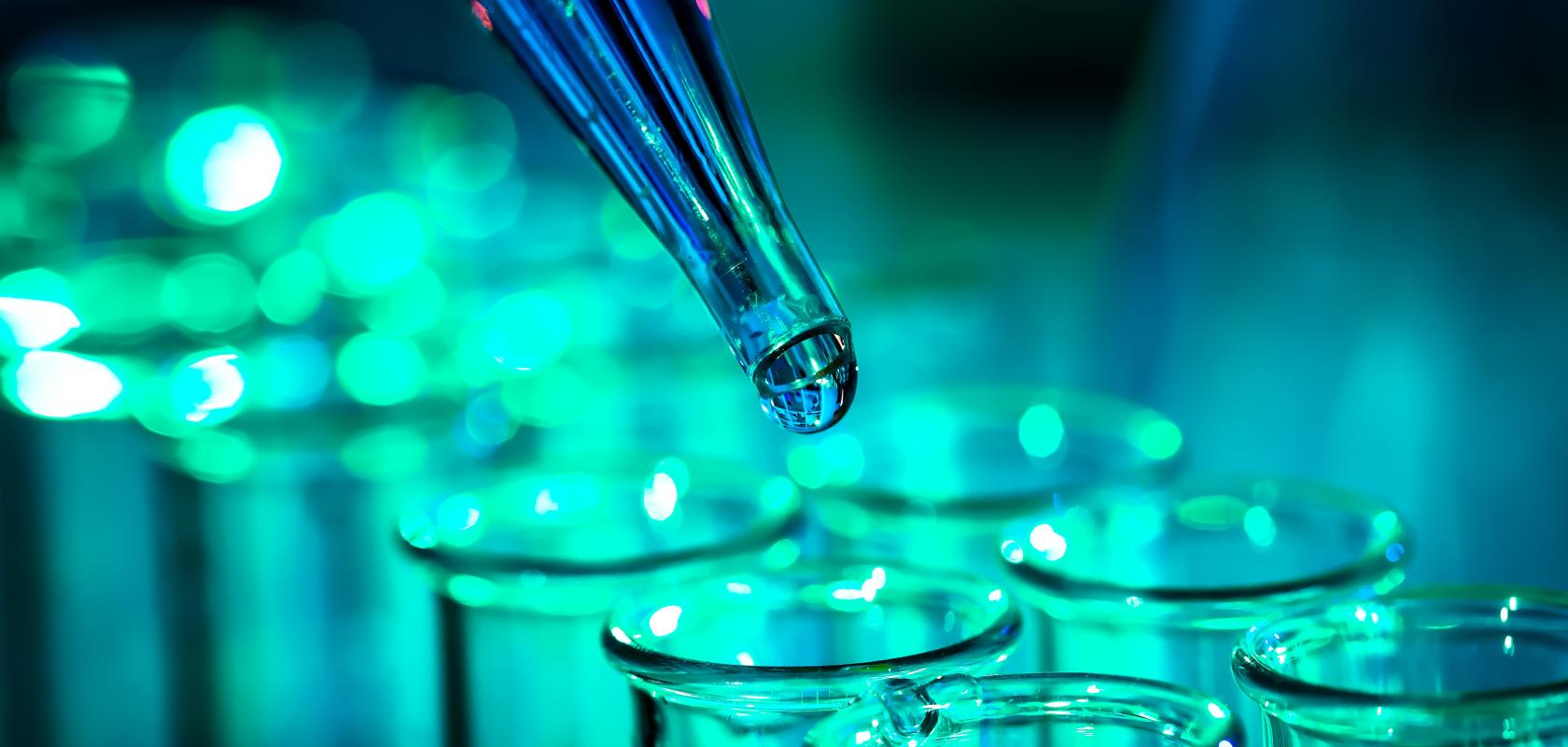
Credit: motorolka/Shutterstock
Ganymede Bio, the cloud infrastructure provider purpose-built for the life sciences and manufacturing industries, and Kytopen, a leader in the development and commercialisation of non-viral transfection tools for genome engineering, today announced a partnership to bring the power of cloud native, structured data to Kytopen’s Flowfect platforms. Under this collaboration, the Kytopen transfection systems will integrate Ganymede’s Lab-as-Code platform for:
Register for FREE to keep reading
Join 12,000+ scientists, engineers, and IT professionals driving innovation through informatics, HPC, and simulation with:
- Insights into HPC, AI, lab informatics & data
- Curated content for life sciences, engineering & academia
- Access to Breakthroughs: real-world computing success
- Free reports & panels, including the Lab Informatics Guide
- White Papers & software updates for smarter research
Sign up now
Already a member? Log in here
Your data is protected under our privacy policy.
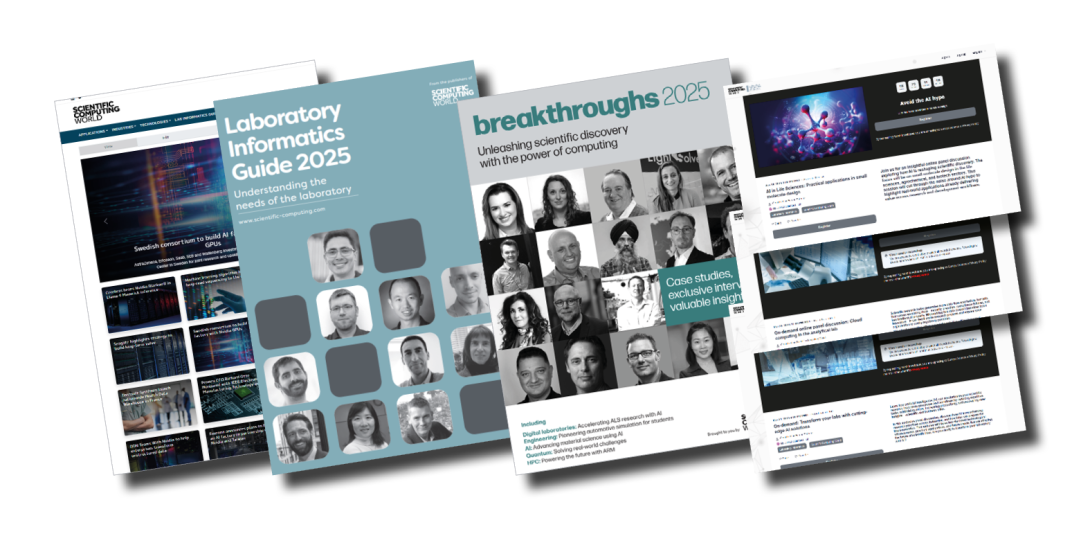